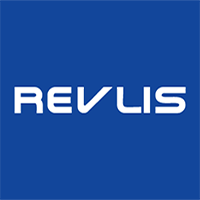
AI-GLU®(AI Core Algorithm)
Starting from electrocardiography, this system integrates unique sensors with a large database to measure various physiological indices, such as blood sugar, glycated hemoglobin, blood pressure, heart rate, etc. Among these, the non-invasive (non-destructive) blood sugar measurement method is particularly notable for its innovative and leading technology. It effectively helps users enhance their health management outcomes, offering a sustainable, highly accurate, and low-burden optimal health management solution.
ECG and Blood Sugar
With the help of artificial intelligence and the Internet of Things, we have been able to calculate physiological values such as blood glucose, glycosylated hemoglobin, blood pressure, and heart rate from ECG electrocardiograms, providing people with comprehensive health management solutions, especially for patients with type 2 diabetes. In 2006, Revlis took the initiative to participate in overseas research projects and discovered the relationship between type 2 diabetes and autonomic nervous dysfunction, so we quickly launched the technical development of non-invasive blood glucose measurement.
The ECG technology uses sensors to receive the human body's electrical signals. When the heart beats, the potential changes generated can show the waveforms of heart rate (heartbeat rate) and heart rhythm (heartbeat rhythm) in ECG, which is the so-called PQRST waveform. The heart rate is based on the spontaneous signal of the heart's sinoatrial node, and combined with the autonomic nerve to control the speed of the heart rate.
Non-Invasive Glucose Monitoring Technology
The principle of non-invasive blood glucose technology is based on the fact that the blood glucose of healthy people rises after eating, thus speeding up the heart rate. By combining the blood glucose concentration with the effect of the glucose utilization of myocardial cells on the heart, different blood glucose conditions can be further deduced by heart rate variability (HRV) and electrocardiogram.
Therefore, physiological parameters such as blood glucose, glycosylated hemoglobin, blood pressure and heart rate can be calculated through AI technology. When the sensor receives the ECG signal, it will send this signal to artificial intelligence machine learning for calculation. With the addition of Revlis’ vast database of records, machine learning will continue to progress, presenting a personalized and interactive technology application.
© 2003 - 2023 Revlis